Mapping the Risk of Spreading Fake-News via Wisdom-of-the-Crowd & MrP
CESifo, Munich, 2024
CESifo Working Paper No. 11138
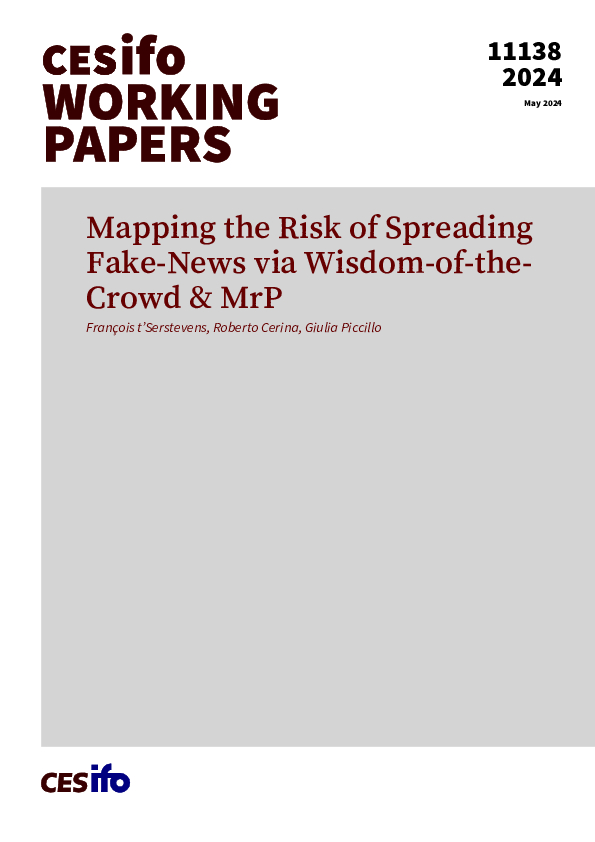
The alleged liberal bias and high costs of fact-checker ratings (Nieminen & Rapeli, 2019) have prevented their ability to limit the spread of fake news. Wisdom-of-the-crowd-based approaches, recognized as a credible alternative to traditional fact-checking methods, have gained prominence for their independence from alleged biases, real-time availability and cost-effectiveness (Allen, Arechar, Pennycook, & Rand, 2021). Instead of fact-checking, this paper utilises a large-scale crowd-sourcing experiment on tweets related to US politics and COVID-19. The objectives of this paper are twofold. First, it develops a method to compute consensus-based post-accuracy indices that are representative of the broader crowds despite their initial non-representative reviewing sample. The computed metrics indicate that Democrat and Republican reviewers have a non-overlapping definition of fake news. Though less accurate than state-of-the-art models, the presented methods provide a deeply explicable, impartial estimate usable for automated content moderation. Second, using the aforementioned accuracy indices, this paper identifies the characteristics of fake news sharers and generates state-wide indices of fake news sharing in the United States. This paper’s findings suggest the tweet author’s political alignment did affect the likelihood of spreading fake news, with Republicans sharing more fake news than Democrats even if an equal number of Republicans and Democrats were in the reviewing sample. The model parameters are subsequently extended to make state-wide fake news-sharing estimates. The resulting state indices and their transparent methodology, provide policymakers with a tool to estimate where fake news policies are most needed.
Empirical and Theoretical Methods
Economics of Digitization